PAST WEBINARS
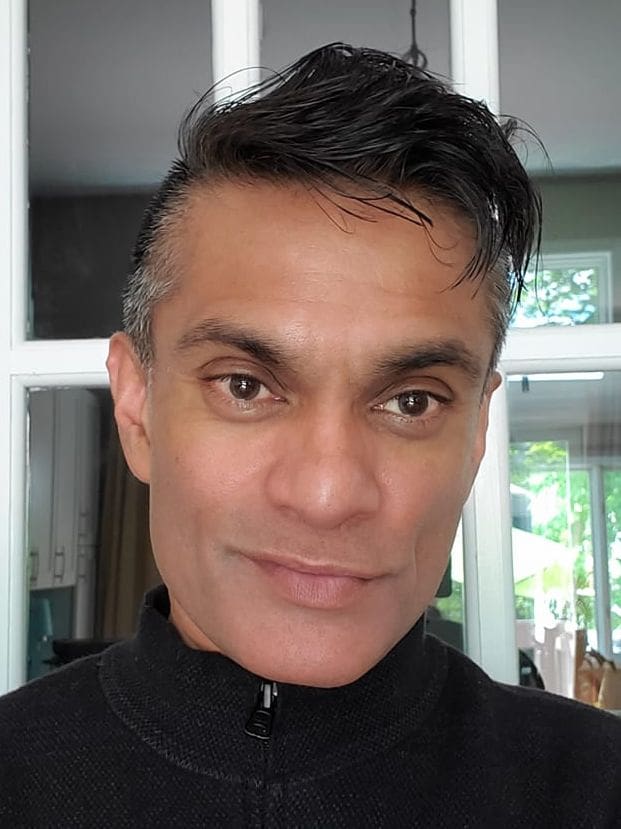
Communicating Science During a Pandemic: Lessons for and from an Epidemiologist
Presented by: Dr. Raywat Deonandan
Date: Tuesday, May 24th, 2022 from 1pm to 3pm EST
Raywat Deonandan is an Epidemiologist and Associate Professor with the Faculty of Health Sciences at the University of Ottawa, as well as a Senior Fellow with Massey College at the University of Toronto. He is a former Chief Scientist with the Canadian federal government, and has received multiple awards for his writing, journalism, and public engagement. During the COVID-19 pandemic, he has been a ubiquitous voice in national and international media.
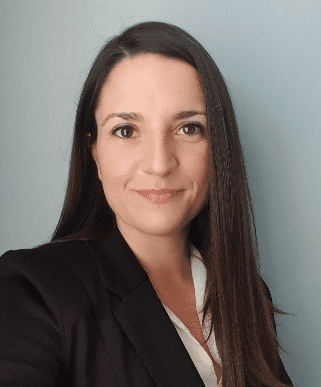
Introduction to Mathematical Models of Infectious Diseases
Presented by: Tiffany Fitzpatrick, PhD
Date: February 9, 2022
As seen throughout the COVID-19 pandemic, infectious disease projections can offer critical insights to inform the selection of optimal public health measures and health systems preparedness. Unlike other diseases, the study of infectious diseases requires a special set of tools, referred to collectively as mathematical models, given the non-independence of events. However, most epidemiologists and biostatisticians receive limited (if any) training in these methods and, consequently, these models are often viewed as “black boxes”. The aim of this workshop, is to demystify these methods and provide participants with an overview of the fundamental concepts related to the use of mathematical models of infectious disease transmission, including the SIR (susceptible – infected – recovered) compartmental model and its variants (e.g. to include additional disease states, vaccination, etc.).
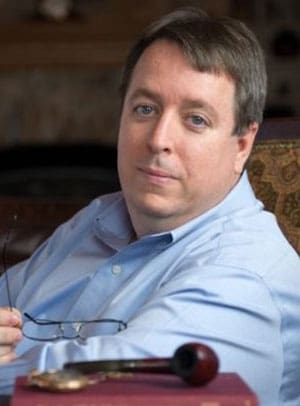
Introduction to Bayesian Statistics Using Stata
Presented by: Chuck Huber
Date: November 17, 2021
Bayesian analysis has become a popular tool for many statistical applications. Yet many data analysts have little training in the theory of Bayesian analysis and software used to fit Bayesian models. This talk will provide an intuitive introduction to the concepts of Bayesian analysis and demonstrate how to fit Bayesian models using Stata. No prior knowledge of Bayesian analysis is necessary and specific topics will include the relationship between likelihood functions, prior, and posterior distributions, Markov Chain Monte Carlo (MCMC) using the Metropolis-Hastings algorithm, and how to use Stata's Bayes prefix to fit Bayesian models.